Today, 60% of all business users are experimenting with foundation models from open source or commercial Large Language Model vendors (LLM Utilities) such as OpenAI (GPT-4; 540BN), Google Enterprise (Palm_2; 1TN), Anthropic (~175BN) and Cohere (52BN). These LLM foundation models are now integral to popular work tools like Microsoft Co-Pilot, Asana, Zoom IQ, Atlassian, Notion AI, HubSpot and many others. With this ubiquity comes the question:
Are your company’s prompt responses and generated code truly private?
The prompts, AI agents and task models your teams build, especially those that automate processes, constitute your company's intellectual property (IP). This AI corpus creates real business value and competitive advantage. It is sensitive, proprietary and not published in public like generative marketing content.
Currently, there's considerable uncertainty about what LLM Utilities are doing with user prompt responses.
Active Learning and Retraining LLMs
The method used for improving ML models is to re-train the models on a training dataset that is updated with the correct inference results. For instance, to improve the performance of a fraud detection ML model, false negatives from the inference on live data will be correctly classified and added to the test dataset upon which the model will be retrained. This requires both the input data to the model and the corresponding inferences of the model. For binary classifiers, both the input and the output (inference) are mandatory. Otherwise, it will not be possible to link the misclassified output to the input. Without them both, it will not be possible to retrain the model to improve its performance.
In NLP, the input (question) can be determined to a high degree of accuracy from analysing the answer. Therefore, it is not always necessary to have the input if an unambiguous and acceptable answer is available. Complex LLMs do not need to store the prompt that produces an acceptable response. They need the response to 'deduce' the prompt and context that produced the response. This holds true for simple instructional prompts used mostly by business users.
To illustrate a use case for this process, an internal company team uses a conversational bot to prompt the LLM Utility for answers. The LLM Utility is connected to data stores and applications. It learns from the user's responses, iterative prompting and confirmation behaviors. The LLM Utility uses this information and behavior to train its data through active learning, including fine-tuning its parameters using prompt responses as inferences for improved accuracy.
Use Case Example
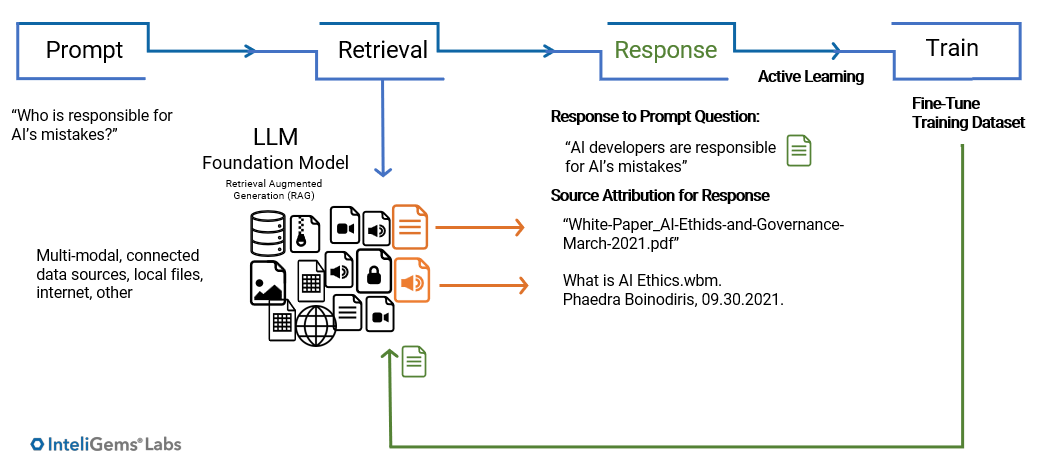
So why should your executive team and board care?
Your LLMs can contain your know-how, prompts, AI models, business processes, trade secrets, knowledge, customer data and financial information. These artifacts are not only ripe for data breaches, but also for accidental ingestion into training datasets that are used to respond to prompts from people outside your organization, including your competitors. This situation also places your organization at risk for non-compliance penalties with regulatory authorities in regulated industries (banking, healthcare, etc.).
_________________________________________________________________________________________________________________________________________________________________________________________________
Federal nonpublic information, including work products, emails, and conversations that are meant to be pre-decisional or internal to GSA, such as financial disclosure information, protected acquisition, controlled unclassified information (CUI), personally identifiable information (PII), and Business Identifiable Information (BII), shall not be disclosed as inputs in LLM prompts as they are not authorized to process or store such data. Such information may be used to expand the training data set and could result in an unintentional breach.
- U.S. General Services Administration, Instructional Letter, David Shive, Chief Information Officer, GSA IT, June 9, 2023
_________________________________________________________________________________________________________________________________________________________________________________________________
If your users are running any prompts that access LLM Utilities via APIs, take pause. You are likely experiencing Prompt Leakage that privacy terms do not cover. LLM Utilities are ingesting your internal and customer prompt responses or inferences for training. Once ingested, inferences cannot be recalled, traced or deleted in a large parameter LLM training data set. This is very difficult to do, if not impossible.
If your LLM is not on-premises or private, your responses can be incorporated into the LLM responses of competitors or outside parties.
LLM Utilities introduce serious privacy, data breach and compliance risks. Business users handling sensitive information want to understand how their queries and data are used and safeguarded. They also need the right LLM environments to match their organization's privacy expectations.
Ambiguous privacy terms and conditions in commercial LLM agreements
One major issue is the ambiguous language and loopholes within the legal privacy terms and conditions (T&Cs) of LLM Utilities. While these T&Cs may project thorough data protection, they do not. Many fall short in complete privacy and ownership of user prompt outputs, inferences, and the results from multi-task agents and task-specific models.
A deeper look into the privacy policies of several LLM Utilities reveals glaring inadequacies. On the surface, these T&Cs assure customers that their data is private and not used for other purposes.
When consents are requested, the T&C language is typically ambiguous, giving LLM Utility providers leeway that might not be immediately apparent. Organizations might be led to believe their prompts are protected and won't be used for training LLMs; the reality is very different. Currently, the nuances in these T&Cs allow LLM Utility providers to use responses in ways that customers did not intend or foresee.
For example, the T&Cs may not cover user prompt output responses or inferences. Inferences are used to fine-tune and train LLMs for accuracy. Several LLM Utilities or third-party vendors go further, failing to recognize customer ownership rights for prompt inputs, outputs and task models.
Can your organization, teams, executives and board trust LLM Utilities?
_________________________________________________________________________________________________________________________________________________________________________________________________
“Every time you disclose data to an LLM, you may be exposing it to broader use in disclosure. Depending on the settings, the LLM may use the data for retraining which means it could end up benefitting your competitors.”
- Jack Dempsey, Security Policy Advisor, Stanford University Cyber Policy Center, May 10, 2023
_________________________________________________________________________________________________________________________________________________________________________________________________
Organisations need more transparency, disclosure and verification to trust LLM Utilities. Two major LLM vendors already face class-action copyright infringement. The T&Cs for LLM Utilities may exploit unsuspecting enterprise users who may be sharing their confidential query results unwittingly, to the competitive detriment of the organisations they work for.
The burden of proof lies with these large LLM Utilities to prove their data privacy policies cover your full scope of authorized usage.
The onus is on LLM Utilities to explain how their models are improved in sufficient detail and proof to allay privacy concerns. Consent for how data is used requires further explanation (What am I agreeing to?). There needs to be verification and recourse when an LLM Utility fails to comply, or a data breach has occurred. Until privacy T&Cs for LLM Utilities prove and state this, the safest path is to consider alternatives, urgently and quickly, lest vital IP is leaked, breached or compliance penalties are incurred.
If you are considering using a commercial LLM Utility for your organization, consider asking these privacy questions as you evaluate the privacy T&Cs of your LLM Utility:
∙ How are prompt outputs used by your LLM Utility?
∙ Are prompt inferences recorded or used to train LLM foundation models?
∙ Is user prompt behavior like response validation tracked?
∙ Can you access AI observability results that prove your LLM Utility provider is not performing active learning on your prompt results?
∙ Can you confirm that your prompt results are not being incorporated into the query results of parties outside your organization?
∙ How does the LLM utility handle data deletion post-termination?
∙ What is the LLM Utility's data retention and breach response policy?
∙ What legal recourse is available in case of data leaks? Note: there is little precedent for enforceability and expensive litigation takes years to settle.
If you are already using an LLM Utility, consider taking these immediate measures to protect your IP and business value:
∙ Prevent employees and contractors from handling sensitive data with public LLMs such as ChatGPT, Bing, and Bard at work or remotely. Opaque privacy combined with the fact that generative AI mimics human behavior, leads users to overshare, unintentionally feeding LLMs or models with sensitive data. Training, while important, should be coupled with automated compliance monitoring.
∙ Review the privacy policies of LLM Utility providers and their third-party tools to identify any potential risks. Consider transitioning to private, domain-specific LLMs for sensitive operations.
∙ Deactivate third-party assistants until LLM Utilities prove your prompt inferences are traceable and not used inappropriately. If the data flows for third-party intelligent assistant tools like Atlassian Intelligence or Zoom IQ are not well-understood or opaque, import their results into a secure, private LLM for further generative AI queries.
∙ Demand transparency in your privacy T&Cs and guarantee enforceability.
∙ Move your domain-specific tasks and GenAI use cases either to an in-house LLM or a private LLM as a service (PlaaS). If it's too challenging to move in-house or expertise is insufficient, consider a PlaaS for domain-specific knowledge vs. general knowledge afforded by a commercial LLM Utility.
∙ Assess current LLM Utility usage by role and permissions.
∙ Identify all sensitive business data that is uploaded, connected or inputted into your LLM.
∙ Harden your privacy infrastructure (see OWASP standards for data leakage and sandboxing).
Conclusion: LLM Utilities – Mitigating a Risky Privacy Affair
Prominent LLM Utility players are integrated into third-party tools such as Asana, Zoom IQ, Otter.AI, Jira, SmartSheet, Salesforce and many others, which many businesses use on a day-to-day basis. These integrations pose alarming risks:
1. Data Privacy Concerns: The privacy policies of these LLMs and third-party tools lack clarity on comprehensive data protection. They don't offer guarantees that prevent LLMs from using prompt response data for training purposes.
2. Inadvertent Data Exposure: Users, often without realization, feed sensitive business data into these commercial LLMs and third-party tools. This action raises substantial concerns about unintentional business data leaks.
3. Lack of Complete Privacy Measures: Currently, there's no foolproof method to make sure that data fed into these models remains private and isn't inadvertently used to improve the models.
4. Prompt Leakage: LLMs hold vast repositories of knowledge, and their responses could inadvertently disclose sensitive data, proprietary algorithms, or confidential details. Such disclosures can lead to unauthorized access, intellectual property breaches, and other associated compliance risks. An example scenario is when an employee, without malicious intent, queries the LLM and inadvertently receives a response containing confidential data.
5. Isolation Concerns: Increasingly, LLMs are being connected to diverse data sources to build comprehensive answering systems, knowledgebases or task agents. Adequate 'sandboxing' or isolation techniques are critical, especially when LLMs can access external resources or sensitive systems. Without stringent isolation, there's a potential risk of unauthorized access or unintended actions by the LLM.
The age of AI and LLMs is undoubtedly exhilarating and creating tremendous advantage. However, the risks associated with LLM Utilities are real and urgent. Organizations must evaluate the potential tradeoffs, data privacy T&Cs, prioritize data security and make informed decisions that safeguard their future. The time to act is now.
The most viable solution is transitioning to private, domain-specific LLMs. Customized for specific business needs, these models ensure data security and privacy without compromising efficiency. They negate the risks of data mingling with external entities, guaranteeing that proprietary information remains confined within organizational boundaries. Such models offer tailored solutions without compromising the security and privacy of business data. Finally, they are increasingly affordable for SMBs and can run as a service, lowering the cost of ownership and the need for scarce data science talent.
By taking these steps, company boards and executives can help protect their companies from the serious risks posed by public LLMs.
References
- Predibase. (2023). "New Research Report: Large Language Models in Production."
- Dempsey, James X. "The security and privacy risks of large language models." Help Net Security, 10 May 2023.
- GSA (General Services Administration). (2023). "Security Policy for Generative Artificial Intelligence (AI) Large Language Models (LLMs)." GSA Instructional Letter CIO IL-23-01.
- Dark Reading. (May, 2023). "Employees Are Feeding Sensitive Business Data to ChatGPT."
- OWASP. (2023). "The OWASP Top 10 for Large Language Model Applications."
- "Generative A.I.’s copyright problem grows as lawsuits pile onto OpenAI, Google, and Meta." Fortune. 12 July 2023.